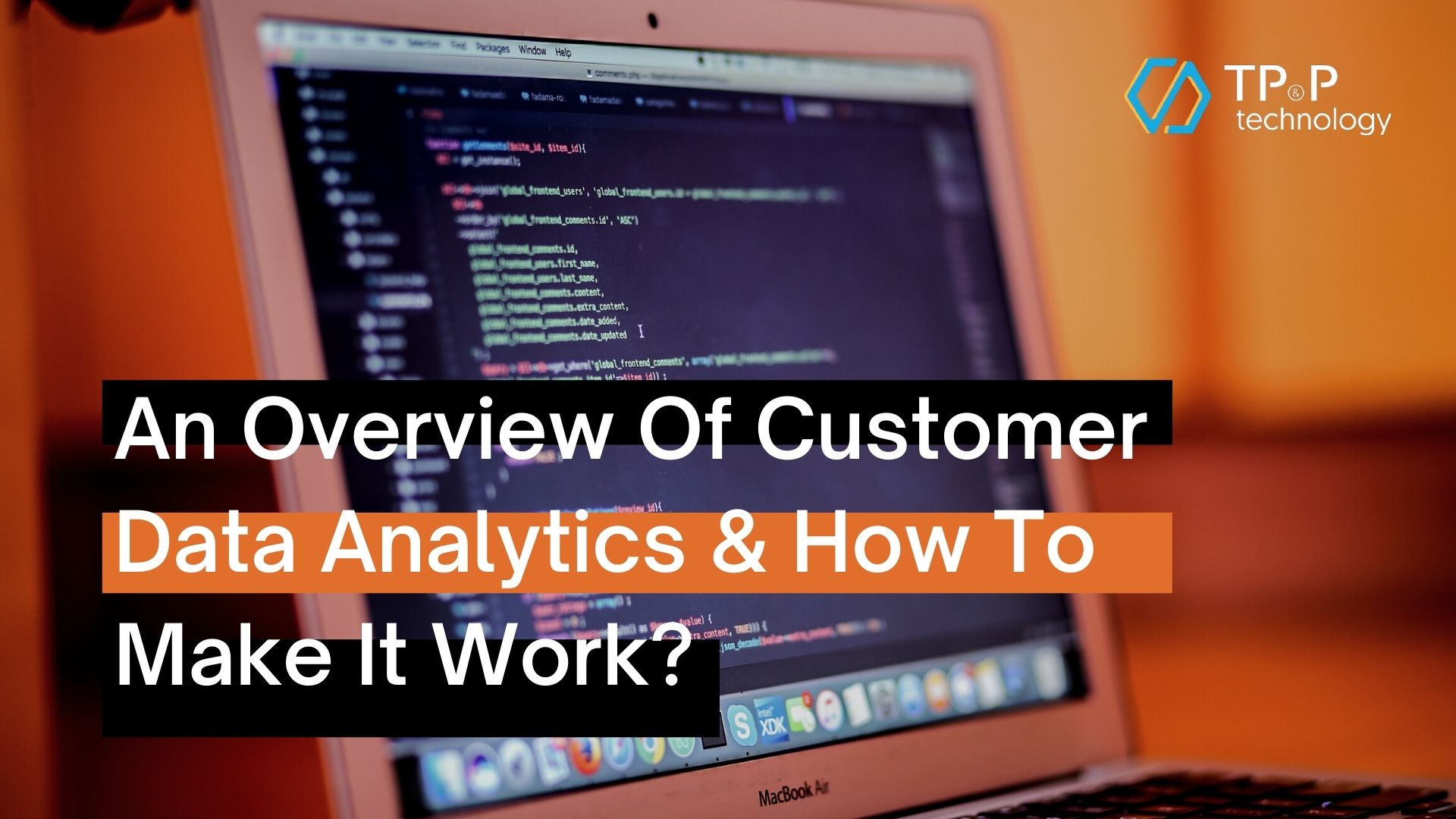
An Overview Of Customer Data Analytics & How To Make It Work?
Modern technology and IoT have enabled customers to tell us what they want with just a few clicks. Too often, however, we are not listening to what they have to say and keep offering the same products and solutions that they have already ignored. If you want to be successful, you, as a business, a solution, and a service provider, have to pay attention to customer feedback and provide them with what they need. You can gain customers’ insights with the rapid speed of today’s analysis technology.
This article will provide you with a complete overview of customer data analytics and four key elements for a successful customer analytics project.
Customer data analytics definition
Businesses understand that a unique customer experience is the competitive advantage that makes them stand out from the competitors, boosts revenue, and builds customer loyalty. And n order to create a unique customer experience, businesses need to know what their customers want. Hence, customer data analytics, also known as customer data analysis or consumer analytics, has become critical.
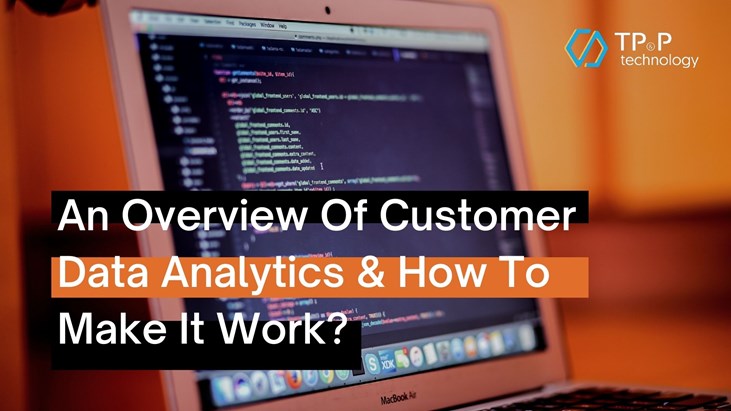
Like the name has indicated, customer analytics collect and analyze customer data to understand customer behavior and preferences to create a business strategy and tactics effectively and automatically form personalized recommendations.
To provide answers to everything-related customers, customer analytics can include different types of business analytics: descriptive analytics, diagnostics analytics, predictive and prescriptive analytics. Businesses can have a 360-degree view of their status quo, find out the business problem's roots, and rely on data science and machine learning to obtain business forecasts and get recommended actions.
Without customer analytics, businesses fail in making decisions in product management, marketing, and sales, leading to unsuccessful products, slow customer acquisition process, business forecasting, which sometimes leads to excessive production or inventory.
4 types of customer data and what they are used for
We divide customer data into 4 main categories to see how businesses in different industries use them.
- Data about services or product use: Manufacturers can see which product and service create the best customer experience and develop a new strategy for the one that doesn’t.
- Transactional data: These data are gathered from retailers and other service providers and allows businesses to identify the sales trends, which stock-keeping units (SKUs) and stores perform best. Hence, businesses can optimize sales and marketing activities at one specific location and estimate growth potential.
- Web behavior data: Businesses can see what their visitors doing on their websites, which pages they visit the most, how deep the visitor’s engagement is, where they are from, etc.
- Data from customer-created texts: Customers tend to leave a review related to a product or service using the review function or via social media post. Companies can study these contents and know their market proposition, what their customers think of their brand or products, solve problems, and identify the opportunity to expand their businesses.
4 areas for successful customer analytics
Marketing and sales strategies
Clear goals and well-defined tactics are needed for a successful customer analytics project. The best-advanced analytics algorithms are not enough to improve business performance unless the whole team understands the strategies and tactics to be implemented and how to use analytics to guide decisions.
First, define your main goal. Do you want to build brand capital, or do you want to create demand? Is the goal to cultivate and manage the potential customers through the system? Does the executive team want to accomplish all of this or more? If they want to achieve all goals and objectives, you’ll need to prioritize all goals and objectives.
Next, determine the strategies used to achieve the goals and what insights will drive the tactics. A brand capital campaign can focus on targeted messaging through mass media and measure results to optimize the marketing mix while measuring demand based on incoming requests, web and mobile traffic, or other steps in the customer journey.
Finally, mapping the results based on model performance and actual measurements back to key performance indicators (KPIs) and financial reporting indicators is essential for understanding the business performance.
Data science expertise
You’ll need an experienced data scientist to navigate the amount of data that your business is processing and generate the detailed results and insights you need. Some of the actions they can take are:
- Treat business challenges as analytical problems
- Perform analysis of a large amount of data
- Ensure that the data provided is relevant to business facts.
- Create training, testing, and data sets validation
- Perform initial baseline modeling
- Choose variables and models to ensure that the correct champions and changers are selected.
Besides, they’ll also determine the root cause and effect to evaluate the model's performance after it is deployed to production for successful customer analysis projects. Knowing how to write the data science/big data algorithm is one of many factors leading to a successful customer data analytics project.
Measuring results
A benchmark or results measurement is indispensable to know whether your customer analysis project is successful. Many marketers have already understood the importance of avoiding last touch attribution when measuring results, as this will increase the impact of the last touch. Multitouch attribution can provide better understanding, as well as the experimental design.
You can also use continuous testing to measure the success, or you can compare champion and challenger performance over a period of time. Also, businesses can understand how customers transition from one business area to another by tracking the customer journey on individual purchases and long-term partnerships.
Ultimately, it is important to measure the results of the core KPIs tracked by business leaders; these KPIs must clearly demonstrate that the financial benefit model is driving business development.
Data and infrastructure
To really understand your customers, it is important to find out all the information available. You'll need to have a significant data infrastructure for large organizations within or close to the petabyte range.
Cloud architecture and code-based infrastructure make it easier to build an analytical lab for model development. Since this data can be susceptible, some of which include confidential information (CI), protected health information (PHI), personally identifiable information (PII), appropriate confidentiality, and masking are necessary.
Customer analytics projects usually require big data and require significant investment in data management and infrastructure. The project team will need time to sort the data, put it in a proper business context, and integrate it into the analysis format.
Conclusion
By leveraging today’s advanced customer analytics tools, your business can win big time since you’ve been turning to a truly customer-centric organization. Read more to know about what big data analytics is and its real-life applications.
Don’t forget to follow our blog post to get yourself up-to-date with the latest technology news. We’re proud to be the top Vietnam software outsourcing company!